
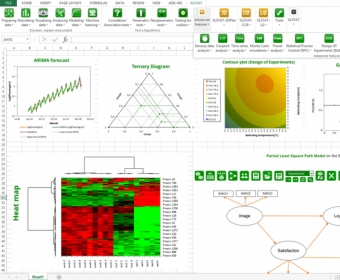
After checking in the source code and testing (see below) we came to the conclusion that the computed coefficients were then reverse standardized, with the inverse of the Artesi transformation, in order to report the coefficients in their natural metric †. Glmnet, by default, standardizes the predictor variables before fitting the model. In the light of the note above, we wanted to adapt the Artesi standardization to the tools we are using. In the edge prediction problem for rephetio, we use the R-package glmnet to perform lasso and ridge regression, in order to perform feature selection while fitting the model. To achieve these two goals, I advise using the most straightforward, simple Agresti method of standardization:Īntoine Lizee Researcher Standardized coefficients & glmnet Standardized coefficients are extremely valuable, mainly to (i) give a meaning to the coefficient affecting a predictor that has no natural metric and (ii) compare effects of predictors reported in different units. With multiple predictors, it is tempting to compare magnitudes of $$\ $$ Conclusion Agresti describes the method as follows (§ 4.5.2 Standardized Interpretations ): Daniel Himmelstein Researcher ApAgresti and I were talking this morning, prior to this discussion, and he arrived at the Agresti method.
